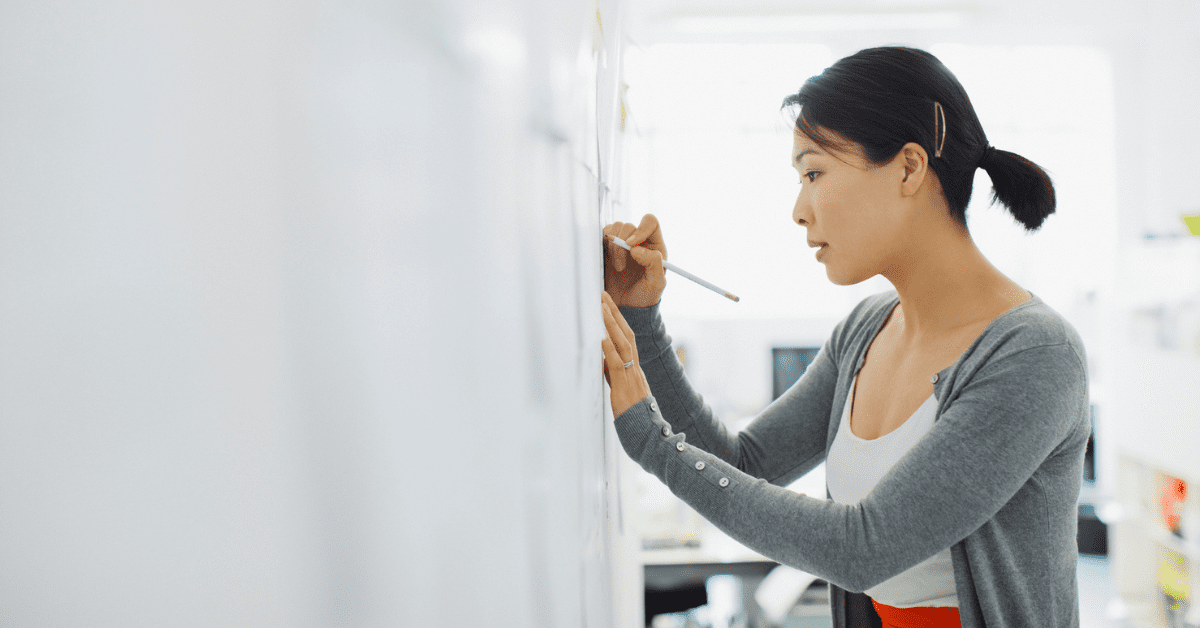
3 Steps to Turn Your Member Data Points Into Action
You have your member data at your fingertips. Now what? It’s time to validate your data and take action based on trends you’ve seen over time.
80% of data work is actually prep work.
We’ve all felt that, whether it’s a massive data science project or a simple monthly report.
“For any data project, you have to clean the data to make sure what you’re working with is accurate and transparent, so that when you show the results you can say exactly what they are and how you achieved them. That way you don’t have to come back and correct anything and answer questions more easily for your team. It’s so important to start at the beginning when you work on data.”
– Nicole Covello, Data Analyst at Wicket
Even if it’s a simple data request on the surface, you have to make sure you can trust the data you’re making decisions with. Because there’s no point in doing data analysis if you can’t take action from it.
That’s what all of this is about.
To make sure you’re prepared to begin your data project, don’t miss Higher Logic’s on-demand webinar series, all about member data. You’ll hear from panelists at Higher Logic, Wicket, and CSAE:
- Session 1: Member Data 101
- Session 2: Exploring Your Data
- Session 3: Turning Insight Into Action
You have all the data at your fingertips. You’ve put it into your member data framework. Now what? It’s time to take action based on trends you’ve seen over time and view the full picture. Today I’m diving into how to make your data trustworthy and actionable — and what to do next once you’ve delivered those insights. Let’s get started:
3 Steps to Validating Your Data
The more mission-critical the decision you’re trying to make, the more important it is to validate your data. You have to know exactly what kind of data you’re using, so that when you present the results, everyone is on the same page.
It’s a time-consuming final step, but it’s actually the most important. You never want someone to say, “Wait, how did we get here?”
“It’s amazing how much time can get absorbed into the details like logic and thought process. But that’s what makes the data accurate. When you’re drilling down on things that have a high level of value, you need additional visibility on those decimal points.”
– Joshua Slyman, Sr. Consultant at Higher Logic
Here are three steps to validating your data:
Step #1: Identify Data Types
First, you need to know exactly what data you’re working with.
If you’re like most associations, you probably have older databases or a mix of places where you store your data. Every time you do a data project at your association, you need to go through that information to understand which data is important, how it was measured, and whether or not that’s different across other data points.
“There are different ways to see if you have any outliers that are going to skew your data, or depending on how the data was formed, understanding if you’re comparing apples to oranges. It can have a really big impact if you don’t go through that testing exercise of understanding what your data looks like at that aggregate level, because you might take action on something that’s flawed.”
– Nicole Covello, Data Analyst at Wicket
You may only have data for 30% of your organization around a specific question. And that’s ok, as long as you’ve made that clear in your analysis — so when it comes time to make a decision, you can be transparent about the quality of the data.
Before you begin, identify the main data types you’re working with:
- Categorical: A list of potential values from a prescribed set. For example, this could be a value like, “Member,” “Lapsed,” or “Non-Member.”
- Date: A period of time. For example, this could be “2020” to indicate their first year of membership.
- Numeric: A percentage, number, or currency. This has a lot more flexibility, but can be something like “$250” for lifetime value.
- Free-form: Open-ended text based on notes, an intake form, or member feedback.
- True/False: Sometimes called a Boolean structure, this is essentially a Yes/No question that your data can answer, like “Subscribed” or “Unsubscribed” to marketing emails, for example.
Step #2: Determine Data Quality
Once you know what type of data is available, you can begin to assess the quality of the data.
One example many associations run into is membership information and renewals. Do you have data you can actually use, or is it a jumble of typed-out cancellation reasons that will take a while to dig through?
“I’m always trying to help understand why members cancel their membership. We used to have an open field where they would just fill out the reason on their own, and using that data to find something meaningful was just really hard. Even if we made the field required, people weren’t willing to input the information if we waited too long to ask. What we did instead was send an exit survey immediately after they told us they weren’t renewing with a set of standard questions, and only one place to put an open response for a little more qualitative feedback. That improved our ability to take action on our renewal rates and made sure it was data we could use.”
– Vasan Selliah, Member Insight and Engagement Manager, CSAE
That’s a great example of why quality data is so important. By turning their membership data into something actionable, CSAE’s team can better make decisions about their marketing program, membership offerings, and take in that feedback to make their association better.
Evaluate your data based on these criteria:
- Completeness: How much data do you have? Is it complete for every field you’re looking at?
- Timeliness: How recent is this data? Have you made major changes to your association since the data was collected? How are you collecting the data in the first place?
- Validity: How does a member interact with this data? What options exist, or is it free-form?
- Consistency: How often is this data point used and filled out? How relevant is this data point to the overall question?
- Integrity: How can you double-check to make sure this data is accurate?
For every type of data, document your validation methods with a template like this one. You can see I’ve carried through Vasan’s example here on the cancellation reasons:
There’s one more method to validate your data using a few common techniques you may have seen in your high school mathematics courses: aggregation.
Step #3: Use Aggregation Methods
Once you know what type of data you have and what quality you’re working with, it’s time for the final step by determining the central tendency of your data.
Measuring the central tendency of your data is a fancy way of saying “Where is most of the data in your data set?” The main metrics to look at are:
- Mean: Synonym for average. You can calculate the mean by taking the sum of all of the values in the data set and dividing by the number of the data set.
- Median: Refers to the middle. If you were to line up every single value from left to right, the median would be the one directly in the middle.
- Mode: Most frequently occurring value in the data set.
- Frequency Distribution: Shows you how spread out your data set is, often through a visualization called a histogram.
- Measurement of Spread: Gives you an idea of the range of values you’re working with, including the minimum, maximum, and any outliers. Often visualized through box-and-whisker-plots.
Aggregation methods help you assess the quality of your data today and how long it will be useful. When it comes to your overall data spread, think back to that membership example:
“I don’t need to have every single member age distributed, but let’s say I have buckets of ten years at a time. For example, if I do a distribution of all of my members that are CEOs, and I see that the majority of them are over sixty years old, that has real consequences in five years as we plan for recruitment, since they’ll be reaching retirement age. That’s very important to know.”
– Beth Arritt, Association Strategist at Higher Logic
BENCHMARK AGAINST YOURSELF
Ultimately, every association is different. It’s great to hear about benchmarks and best practices for context, but the best place to find answers is within your own data.
You need to benchmark against yourself. What’s performing well? What’s not? What do you need to change?
You can find these answers in your data.
When you build your own structure, you give yourself so much more flexibility to change your activities and actions on the fly, since it’s your initiatives that are going to move the needle on those benchmarks.
When you’re ready to dive into validating your data, follow these steps:
- Think about the level of accuracy you’ll need
- Create a plan to direct your validation efforts
- Work validation time into planning
- Document the results of your validation
- Be prepared to modify your plan
- Don’t reinvent the wheel
Want to learn more? Check out the Let’s Talk Member Data webinar series.